Disclaimer: The F1 FORMULA 1 logo, F1 logo, FORMULA 1, F1, FIA FORMULA ONE WORLD CHAMPIONSHIP, GRAND PRIX and related marks are trademarks of Formula One Licensing BV, a Formula 1 company. All rights reserved. I’m just a dude in a basement with too much time on my hands.
This is a multi-part series.
Part 1 – this article
Part 2 – Imola / Portimão
Let’s take out the first issue out of the way – I know it’s way too late to make predictions about the Bahrain race. I’ve been sitting on this blog post for quite some time, so I’m way behind. There is one more about Imola and Portimão incoming and hopefully by the Spanish Grand Prix will be all caught up.
Why am I gonna focus on tyres? (for now)
For the start, I will focus on the tyre strategy. Since refuelling was banned in 2009, choice of tyres and when to stop to change them has been a crucial part of the race strategy. In modern Formula 1 there’s only one tyre supplier – Pirelli. There are 5 compounds for dry condition (C1 is the hardest, and C5 the softest) and two types of tyres for wet conditions – “Wet” for heavy standing water on the track and Intermediate for the track that’s drying. For each race Pirelli picks three dry compounds for the teams and assign them more human-readable names – Hard (marked with white stripes), Medium (marked with yellow stripes) and Soft (marked with red stripes). So for example for Bahrain this year C2 was marked Hard, C3 – Medium and C4 – Soft. C1 and C5 were not used during that race.
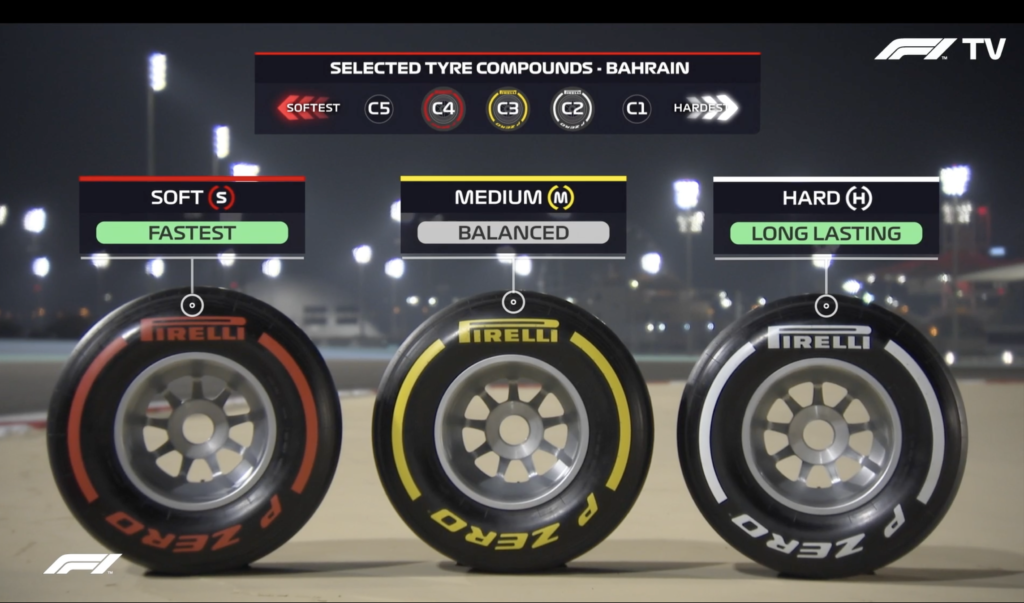
For different races, those options may be different (for example in Portimão last week C1 was Hard, C2 – Medium, C3 – Soft). Pirelli makes that decision depending on how abrasive and destructive for tyres is the particular track. For each racing weekend in 2021 teams receive 8 red (Soft) sets of tyres, 3 yellow (Medium) and 2 white (Hard) sets for each card and are free to use them as they wish, but they need to use at least two compounds in the race. This rule is not enforced if there are wet conditions. For those occasion the team receives 4 sets of Intermediate and 3 sets of Full Wet tyres for each car.
Generally softer tyres have better grip (and hence cars can go through corners at higher sped → lower lap times), but lower durability and hard tyres have good durability but worse grip. They also have different operating temperatures, so it’s easier to overheat the soft tyres and more difficult to put the hard tyres into good operating temperatures. Harder tyres usually provide slower lap times, but you can cover longer distance in the race. It’s a balancing act – you can use three sets of softer tyres and hope that with better lap times you can cover the 20-30 seconds time you needed for additional tyre change. Or do only one stop on slower/harder compounds. This usually works better on tracks where it’s harder to overtake – even if you’re slower because of the harder compound, the faster car may not be able to overtake you. We could talk about it for hours, but I’ll introduce more nuances as we go along. This is enough nerd talk about rubber for now.
ML.NET
I’m going to use ML.NET in this series of posts. I have some experience with it, but I want to learn more about the whole ecosystem while doing some fun project. Training, inference, deploying models, visualizing data – basically making a working “product” and seeing what tools we can use along the way. For context, I generally have experience building machine learning – powered products in Python frameworks, and I also have years of knowledge working in .NET projects. I want to connect those worlds. And even though I have some previous knowledge, I’m going to try to jump into it like I know nothing and make it a learning experience for you and me.
ML.NET is an open-source cross-platform machine learning library built on .Net. It implements several type of ML algorithms for solving few most common problems. It doesn’t have the flexibility of TensorFlow, but the learning curve is much more approachable, and it allows you to be fairly productive pretty quickly without investing a lot of maths knowledge. Is it the responsible approach? Probably not in all cases. You should always have a good understanding of tools you use to not hurt yourself or others.
We’re solving a regression problem
ML.NET approach is: start first with what kind of problem you’re solving. When trying to predict a specific numeric values (number of laps that tyre will be used), based on past observed data (tyre stint information from previous races), in machine learning this type of prediction method is called regression.
Linear regression is a type of regression, where we try to match a linear function to our dataset and use that function to predict new values based on input data. A classic example of linear regression is predicting prices of a flat based on its area. It’s a fairly logical approach – the bigger the flat the more it costs.

So on the image above you can see a simple chart with input data (green dots), the linear function that we matched to the data. And the red dot is our prediction for a new data point. Matching that function to the data set is the “learning” part, sometimes also called “fitting”. Predicting new value from that function is “inference”.
The input values are called “features”, and the outputs we have in our learning data sets are called “labels”. With one feature we can visualize that data in two dimensions, but the more features the visualizations gets a bit out of control of our 3-dimensional minds. Fortunately maths much more flexible. Initially I’ll be using 11 features for our dataset to solve this problem.
My dataset
So where do I get the data? I’m using what’s publicly available, and hence my results will be much less precise than what teams can do. But let’s see where that will lead us.
I decided, as a first task, to predict a “stint” length for each driver on each type of tyre. Stint is basically a number of laps driver did on a single set of tires. If we have one change of tyres in the race, we have two stints – one from the start to the tyre change, and the second from the tyre change to the race finish. Two tyre changes – three stints. You get the grips of it. Sometimes a stint is finished with less positive outcome, like accident, or retirement because of technical issues. Sometimes it extends too much and finish with a tyre puncture. Usually teams will try to get max out of the tyres, but not overdo it.
I took data from charts like this and then watched race highlights or in some cases full races to figure out why some stints were shorter than expected. On the image below from this year’s Bahrain GP you can see that most drivers were doing 3 stints, or to use Formula1 lingo – they were on “two stop strategy”. And for some drivers the lines are shorter, because their races finished with one of those less-favourable outcomes.

To make reasonable predictions, I focus only the period from 2017 to 2020. Why this period? 2017 was the last time we had major changes in aerodynamics and tyre constructions. And even though there were some minor modifications since then, the cars are in principle similar, and they abuse the tyres similarly. We also have many drivers overlapping, so we can find some consistency in their style of driving.
I also picked only races on the same track for this first approach. There are few reasons. I’m removing the variable of different track surfaces. The races are the same length in this period, so I can focus on predicting stint lent in “laps” and don’t need to worry about calculating it from distance covered.
Each row in the dataset is one stint. I collected features as listed below:
- Date – mostly for accounting reasons, but maybe in future we could extract time of year for some purpose.
- Track – different track, different surface properties impact tyre wear (although for now it’s only Bahrain)
- Layout – sometimes racing uses different layout on the same track (for example Bahrain has 6 layouts, out of them 3 were used in Formula 1 since 2004, but mostly irrelevant for the 2017-2020)
- Track length – will be useful when predicting covered distance instead of laps for future races
- Team – different teams approach strategy a bit differently (for example priority for different drivers) – may be relevant in future
- Car – different cars have different aero properties and “eat-up” tyres differently
- Drivers – different driving styles, different levels of tyre abuse – for example Lewis Hamilton owes several of his victories to great tyre management
- Tyre Compound – which tyre compounds were used during that sting. I use the C1-C5 nomenclature as on different races “Soft” can mean different things, and C1-C5 range is universal (apart from year 2017, but I mapped it to current convention)
- Track Temperature – warmer surface allows quicker activation of tyres, but also quicker wear; too cold is not good either, because tyres can be susceptible to graining, which also lowers their lifespan.
- Air Temperature – not sure, but I had access to data, so decided to keep it
- Reason – why the stint ended – for example Just regular Pit-stop, End of Race, Red Flag, Accident, DNF
- Number of laps covered in a stint – this will be my “label”, so what we’re training to be able to predict
The code
The code, minus the dataset, is available on my GitHub.
As I mentioned, my approach will be like I’m completely new to ML.NET. We already established that we’re solving a regression problem. So I copied the sample regression project that predicts rental bikes demand and made minimal changes to the code.
First, I have a single dataset. In machine learning you generally want to have a training set – to train, and a testing set to test your hypothesis on data. Testing data shouldn’t be process by algorithm. It’s a bit like taking a test knowing sample questions, but not having the exact question that will be on the test. Bike rental example has already those datasets divided, but I had to add that bit in my case.
// Load data
var data = mlContext.Data.LoadFromTextFile<TyreStint>(DatasetsLocation, ';', true);
// Divide dataset into training and testing data
var split = mlContext.Data.TrainTestSplit(data, testFraction: 0.1);
var trainingData = split.TrainSet;
var testingData = split.TestSet;
Next I build the calculation pipeline. It’s basically the order of transformation we want to do on the data. I just replaced field names from the example code with the fields I use in my dataset. You can notice quite a lot of “OneHotEncoding”. This is an operation that indicates this field is a categorical data. So for example, we don’t want to assume that “Alfa Romeo” is better than “Red Bull” because it sits higher in alphabetical order. We also normalize the values for Numerical data, so they’re easier computationally for the fitting algorithm.
// Build data pipeline
var pipeline = mlContext.Transforms.CopyColumns(outputColumnName: "Label", inputColumnName: nameof(TyreStint.Laps))
.Append(mlContext.Transforms.Categorical.OneHotEncoding
(outputColumnName: "TeamEncoded", inputColumnName: nameof(TyreStint.Team)))
.Append(mlContext.Transforms.Categorical.OneHotEncoding
(outputColumnName: "CarEncoded", inputColumnName: nameof(TyreStint.Car)))
.Append(mlContext.Transforms.Categorical.OneHotEncoding
(outputColumnName: "DriverEncoded", inputColumnName: nameof(TyreStint.Driver)))
.Append(mlContext.Transforms.Categorical.OneHotEncoding
(outputColumnName: "CompoundEncoded", inputColumnName: nameof(TyreStint.Compound)))
.Append(mlContext.Transforms.NormalizeMeanVariance
(outputColumnName: nameof(TyreStint.AirTemperature)))
.Append(mlContext.Transforms.NormalizeMeanVariance
(outputColumnName: nameof(TyreStint.TrackTemperature)))
.Append(mlContext.Transforms.Categorical.OneHotEncoding
(outputColumnName: "ReasonEncoded", inputColumnName: nameof(TyreStint.Reason)))
.Append(mlContext.Transforms.Concatenate
("Features", "TeamEncoded", "CarEncoded", "DriverEncoded", "CompoundEncoded",
nameof(TyreStint.AirTemperature), nameof(TyreStint.TrackTemperature)));
So far so good, let’s now train that pipeline with our test data. It looks surprisingly simple. I didn’t mess with the algorithm or the parameters of it. Just took the same regression algorithm used in Bike Rental examples. There will be time for some optimizations later.
var trainer = mlContext.Regression.Trainers.Sdca(labelColumnName: "Label", featureColumnName: "Features");
var trainingPipeline = pipeline.Append(trainer);
// Training the model
var trainedModel = trainingPipeline.Fit(trainingData);
So is our model good to make some predictions? Let’s try it out:
var predictionEngine = mlContext.Model.CreatePredictionEngine<TyreStint, TyreStintPrediction>(trainedModel);
var lh1 = new TyreStint() {
Team = "Mercedes", Car = "W12", Driver = "Lewis Hamilton",
Compound = "C3", AirTemperature = 20.5f, TrackTemperature = 28.3f, Reason = "Pit Stop"};
var lh1_pred = predictionEngine.Predict(lh1);
var mv1 = new TyreStint() {
Team = "Red Bull", Car = "RB16B", Driver = "Max Verstappen",
Compound = "C3", AirTemperature = 20.5f, TrackTemperature = 28.3f, Reason = "Pit Stop" };
var mv1_pred = predictionEngine.Predict(mv1);
I run two example runs for top drivers. Lewis Hamilton got 19.46 laps on C3 tyre (“Medium” for Bahrain) and Max Verstappen – 14.96 lap. First of all values are reasonable – they’re not outrageously low or high. They also show what’s generally tends to be true – that Lewis Hamilton is better at tyre management than Max Verstappen. But they most likely include the fact, that Max was doing very poorly in recent years in Bahrain and on average had shorter stints because of DNFs.
So how good did we do?
So here are our predictions vs real stints of drivers. If the drivers took more than one stint on the compound, I listed all of them. If the driver didn’t use that tyre, the Actual column will be empty.
Driver | Compound | Prediction | Actual |
---|---|---|---|
Lewis Hamilton | C2 | 26,78 | 15; 28 |
Lewis Hamilton | C3 | 19,81 | 13 |
Lewis Hamilton | C4 | 16,18 | – |
Valtteri Bottas | C2 | 28,53 | 14; 24 |
Valtteri Bottas | C3 | 21,56 | 16; 2 |
Valtteri Bottas | C4 | 17,92 | – |
Max Verstappen | C2 | 22,27 | 17 |
Max Verstappen | C3 | 15,31 | 17; 22 |
Max Verstappen | C4 | 11,67 | – |
Sergio Pérez | C2 | 26,37 | 19 |
Sergio Pérez | C3 | 19,41 | 2; 17; 18 |
Sergio Pérez | C4 | 15,77 | – |
Lando Norris | C2 | 25,62 | 23 |
Lando Norris | C3 | 18,65 | 21 |
Lando Norris | C4 | 15,01 | 12 |
Daniel Ricciardo | C2 | 29,19 | 24 |
Daniel Ricciardo | C3 | 22,22 | 19 |
Daniel Ricciardo | C4 | 18,58 | 13 |
Lance Stroll | C2 | 23,35 | 28 |
Lance Stroll | C3 | 16,39 | 16 |
Lance Stroll | C4 | 12,75 | 12 |
Sebastian Vettel | C2 | 23,15 | 31 |
Sebastian Vettel | C3 | 16,18 | 24 |
Sebastian Vettel | C4 | 12,54 | – |
Fernando Alonso | C2 | 32,52 | 3 |
Fernando Alonso | C3 | 25,55 | 18 |
Fernando Alonso | C4 | 21,91 | 11 |
Esteban Ocon | C2 | 32,11 | 24 |
Esteban Ocon | C3 | 25,14 | 18 |
Esteban Ocon | C4 | 21,50 | 13 |
Charles Leclerc | C2 | 29,28 | 24 |
Charles Leclerc | C3 | 22,31 | 20 |
Charles Leclerc | C4 | 18,67 | 12 |
Carlos Sainz | C2 | 26,97 | 19 |
Carlos Sainz | C3 | 20,01 | 22 |
Carlos Sainz | C4 | 16,37 | 15 |
Pierre Gasly | C2 | 32,93 | 15; 13 |
Pierre Gasly | C3 | 25,96 | 4; 20 |
Pierre Gasly | C4 | 22,32 | – |
Yuki Tsunoda | C2 | 30,88 | 18; 23 |
Yuki Tsunoda | C3 | 23,91 | 15 |
Yuki Tsunoda | C4 | 20,27 | – |
Antonio Giovinazzi | C2 | 26,41 | 18 |
Antonio Giovinazzi | C3 | 19,44 | 12; 25 |
Antonio Giovinazzi | C4 | 15,806 | – |
Kimi Räikkönen | C2 | 25,65 | 16 |
Kimi Räikkönen | C3 | 18,68 | 13; 27 |
Kimi Räikkönen | C4 | 15,04 | – |
Nikita Mazepin | C2 | 25,92 | – |
Nikita Mazepin | C3 | 18,95 | – |
Nikita Mazepin | C4 | 15,31 | – |
Mick Schumacher | C2 | 25,92 | 22 |
Mick Schumacher | C3 | 18,95 | 14; 19 |
Mick Schumacher | C4 | 15,31 | – |
George Russell | C2 | 21,46 | – |
George Russell | C3 | 14,49 | 23; 19 |
George Russell | C4 | 10,85 | 13 |
Nicholas Latifi | C2 | 22,00 | – |
Nicholas Latifi | C3 | 15,04 | 18; 19 |
Nicholas Latifi | C4 | 11,40 | 14 |
So predictions are actually relatively reasonable. Those are not numbers that do not make sense. The model figured out that the C2 (Hard) tyres are lasting longer than C3 (Medium) and C4 (Soft). The numbers seem to be higher for drivers with better tyre management skills. They of course do not match the real numbers from the race, because there’s a lot of other things happening in the race. Crashes, retirements, reacting to strategy of other teams. Those are all things that we’ll need to somehow include in the future, but that’s not a problem for today. Overall I feel good about it. I think we have a decent model.
What does the metrics say?
But do we? Running some metrics that ML.NET have built in…
var predictions = trainedModel.Transform(testingData);
var metrics = mlContext.Regression.Evaluate(predictions, labelColumnName: "Label", scoreColumnName: "Score");
Console.WriteLine($"*************************************************");
Console.WriteLine($"* Metrics for {trainer.ToString()} regression model ");
Console.WriteLine($"*------------------------------------------------");
Console.WriteLine($"* LossFn: {metrics.LossFunction:0.##}");
Console.WriteLine($"* R2 Score: {metrics.RSquared:0.##}");
Console.WriteLine($"* Absolute loss: {metrics.MeanAbsoluteError:#.##}");
Console.WriteLine($"* Squared loss: {metrics.MeanSquaredError:#.##}");
Console.WriteLine($"* RMS loss: {metrics.RootMeanSquaredError:#.##}");
Console.WriteLine($"*************************************************");
…gives following output:
*************************************************
* Metrics for Microsoft.ML.Trainers.SdcaRegressionTrainer regression model
*------------------------------------------------
* LossFn: 103,16
* R2 Score: -0,26
* Absolute loss: 7,99
* Squared loss: 103,16
* RMS loss: 10,16
*************************************************
I’m not going to dig into what all of those metrics mean and just focus on the R2 Score, also known as Coefficient of determination. The better our function fits the testing data, the closer that value is to 1. 0 is bad. Negative is catastrophically bad. So even though some of our values looks reasonable, it’s probably accidental at this point. We’ll explore possible reasons for that and other metrics in future posts. Tyres vs Michał 1:0.
What’s next?
In the next instalment we’ll look at two races of Imola and Portimão. They will be tricky to get any reasonable results, because both of them will be running for the second time in recent years. But this will be a good occasion to extend our model and dataset to include other tracks. We’ll no longer be using laps as our label, because different tracks have different lengths. We’ll use total distance covered and calculate the number of laps out of it.
We should also look into removing some irrelevant data, like stints ended with crashes or done purely to beat the fastest lap (it’s a thing, but a long story to explain).
At some point we’ll also have to take a crack and why those metrics are so bad, and try different regression optimizers and different hyperparameters.
Until next time!
Part 2 – Imola / Portimão